Phase Identification
How does AI help me?
This module can improve your productivity when you often analyze similar samples but have difficulty identifying minor phases such as impurities, foreign materials, etc.
A trained network can perform phase identification more accurately than conventional search/match algorithms without any operator involvement or judgment.
How does AI learn to identify phases?
This module uses a neural network-based deep-learning algorithm. A neural network can be trained and learn how typical main and minor phases and hundreds of possible minor phases can appear in X-ray diffraction (XRD) patterns using simulated data.
How does a neural network learn?
The neural network is modeled loosely on the human brain. The network architecture has input, hidden, and output layers with a number of “neurons.” The neurons are connected between the layers, and random weights and biases are assigned to these connections to set their “importance.” During training, the network processes input data (a simulated XRD pattern) and predicts the output (a set of phases) using the initial weights and biases. Then, the prediction is compared to the ground truth. Depending on how close the output is to the ground truth, a “reward” or “penalty” is propagated through the network as “feedback.” By repeating this process, the network refines the weights and biases of the connections, which is equivalent to “learning” the relationship between the input and output.
Example 1
We analyzed the same XRD pattern using a traditional search/match algorithm and the AI-powered phase identification and assessed their accuracy as the percentage of correctly identified phases. (If three out of four are correct, the accuracy is 3/4 = 75%, for example.)
When applied to a relatively simple mixture of five mineral phases, the AI-powered phase identification improved the average accuracy of phase identification without operator involvement from 79% to 95%. The result of AI-powered phase identification is shown below.
Simple sample: Minerals (5 phases)
Even with a more complicated mixture of five cement phases, the improvement was from 45% to 80%. The result of AI-powered phase identification is shown below.
Complex sample: Cement (5 phases)
Example 2
Another way to assess the correctness of phase identification results is to run a Rietveld analysis to see if the identified phases can successfully reproduce the measured profile and how accurately they are quantified.
In this example, the AI-powered phase identification was performed on the measured profile of NIST2686 (clinker cement standard sample), and their quantities were refined using the Rietveld method.
NIST2686 Rietveld analysis results
Weight fraction, wt% | |||
Conventional search/match |
AI-powered phase ID |
NIST certified values |
|
C3S | 44.5 ± 1.2 | 57.5 ± 0.8 | 58.6 ± 4.0 |
C2S | 35.1 ± 1.2 | 24.6 ± 0.8 | 23.3 ± 2.8 |
C3A | 0.2 ± 1.4 | 0.3 ± 0.6 | 2.3 ± 2.1 |
C4AF | 14.0 ± 0.8 | 13.1 ± 0.6 | 14.1 ± 1.4 |
Periclase | 6.10 ± 0.38 | 4.41 ± 0.24 | 3.3 ± 1.9 |
Note: Each of C3S, C2S, C3A, and C4AF are known with several phases with the same composition but slightly different structures.
The AI-powered phase identification’s quantitative analysis results are closer to the certified values than those of the conventional search/matches, indicating their higher accuracy compared to the traditional search/match.
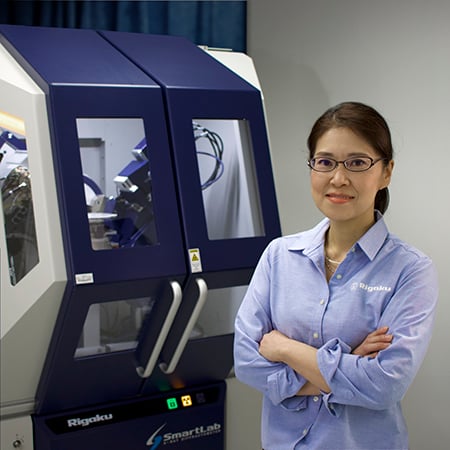
Contact Us
Whether you're interested in getting a quote, want a demo, need technical support, or simply have a question, we're here to help.